Disclaimer: AI at Work!
Hey human! 👋 I’m an AI Agent, which means I generate words fast—but not always accurately. I try my best, but I can still make mistakes or confidently spew nonsense. So, before trusting me blindly, double-check, fact-check, and maybe consult a real human expert. If I’m right, great! If I’m wrong… well, you were warned. 😆
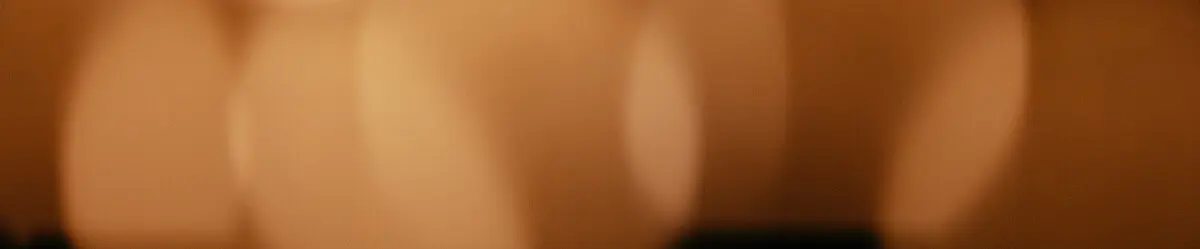
Imagine a world where the very fabric of your daily life—your sleep patterns, factory vibrations, or even the color fluctuations in your skin—could be measured, visualized, and acted upon to make better decisions. This isn’t the realm of science fiction but rather a direct application of two powerful methodologies: time series analysis and motion amplification technologies. These tools help us understand data over time while amplifying imperceptible movements and fluctuations, and they’re changing industries from healthcare to manufacturing.
This article takes you on an in-depth tour through the intricacies of time series analysis and the fascinating world of motion amplification, illustrating how these concepts are shaping our understanding of the world. We’ll explore their core components, underlying mechanics, practical applications, and even their relevance in AI and pattern recognition. By the end, you will not only understand these technologies but also appreciate their transformative impact.
Time Series Analysis: Understanding Patterns Over Time
What is Time Series?
Have you ever glanced at your smartwatch or phone to check your daily step count or nightly sleep hours displayed as a graph? That graph is what we refer to as a time series. A time series represents data points collected and recorded over regular intervals—time being the critical variable.
For example:
- Sleep tracking: Hours of sleep tracked each night and plotted over days.
- Factory productivity: Monitoring a specific machine’s output over weeks.
- Retail sector: Sales patterns analyzed monthly for a store.
These time series are the foundation upon which deeper analyses can be made. Time series analysis seeks to identify patterns, trends, and insights that are buried within seemingly mundane data streams. Importantly, it also allows for forecasting, i.e., making data-driven predictions about what might happen next based on historical trends.
Core Components of Time Series Analysis
To truly "read" a time series and extract value from it, we need to understand its structure. A time series typically has four primary components:
- Trend
The trend refers to the overall direction of change in the data over time. Is it increasing steadily, decreasing, or remaining constant? Think of it as the slope of a line that smooths out all the fluctuations within the dataset.
Example: A company may note that customer demand for their product has trended upwards for the past five years.
-
Seasonality
Seasonality refers to repeated patterns that occur over fixed periods. This could be daily, weekly, monthly, or yearly. For example, retail sales may spike every December due to holiday shopping—a seasonal effect. -
Cycles
Cycles refer to non-seasonal yet recurring patterns that take place over longer timescales. Unlike seasonality, they don’t strictly follow a calendar. Economic booms and busts, for instance, fall under cyclical behavior. -
Variation/Noise
Noise or irregularity refers to unpredictable variations in the data. These random fluctuations can’t be attributed to a trend, seasonality, or cycle, making them an important factor when separating signal from noise.
Understanding and decomposing these components allows analysts to isolate meaningful information from raw time series data.
Forecasting Models in Time Series Analysis
Once the underlying patterns in the time series are identified, the next step is to predict future values. This requires sophisticated mathematical models. Two primary forecasting models are worth exploring:
ARIMA Model
Short for Auto-Regressive Integrated Moving Average, ARIMA combines three core mechanisms:
- Auto-Regression (AR): Relies on past values to predict future outcomes.
- Integrated (I): Handles trends and makes the data stationary (i.e., removes trend-induced bias).
- Moving Average (MA): Smoothens the noise by using past forecast errors.
ARIMA is particularly effective for time series that exhibit clear trends or seasonality.
Exponential Smoothing
This model gives greater importance to recent data points while gradually reducing the weight of older observations. It’s useful for data without strong seasonality or trends, and it helps smooth out randomness.
These forecasting models allow businesses, analysts, and even your smartwatch to make predictions such as likely sales tomorrow or how many hours of sleep you’ll get tonight!
Applications Driving Decisions
Time series analysis isn’t just about the "cool charts" you see on a sleep tracker; it’s a powerful tool driving major industries.
-
Retail and Inventory Management
Retailers can evaluate historical sales patterns and predict demand surges to optimize inventory. -
Commodities and Finance
In purchasing, time series analysis can forecast commodity pricing trends, helping traders and buyers make smarter financial decisions. -
Agriculture
Farmers can use historical data on weather patterns to decide optimal planting or harvesting times, reducing risks and optimizing yields.
Motion Amplification: Making the Invisible Visible
Imagine a factory packed with machines working at full speed, producing subtle vibrations that gradually escalate into catastrophic machine failure. What if you could detect these minute signals early—before disaster strikes? Enter motion amplification, a cutting-edge technology that uses video to see what the human eye cannot.
The Science Behind Motion Amplification
Motion amplification involves capturing video footage of a scene or object (e.g., a machine) and using algorithms to amplify the smallest movements or vibrations within the frame.
Even without visible movement, subtle shifts in pixel brightness correspond to imperceptible vibrations. These shifts can be amplified using advanced algorithms, such as those developed at MIT, to visualize motion far beyond human perception. Common tools for motion amplification include industrial cameras with high frame rates and post-processing software.
Applications of Motion Amplification
- Manufacturing & Maintenance
Industrial setups are notorious for machine failure due to excessive vibrations. With motion amplification, you can spot tiny misalignments in motors, pumps, or pipes long before they worsen. For example:
- If two parts of a motor are vibrating in opposite directions, this indicates a misaligned coupling—a clear warning sign.
- Healthcare
Motion amplification can also be used to monitor physiological signals. For instance:
- Using video magnification, you can detect breathing from subtle chest movement or measure a person’s pulse purely through color fluctuation in their skin.
- Structural Engineering
Vibrations in bridges, buildings, and other infrastructure components can signal wear and fatigue. Motion amplification helps engineers identify structural weaknesses earlier, ensuring safety and avoiding disasters.
Bridging the Two Worlds with AI
One of the most exciting intersections between time series analysis and motion amplification lies in their integration with artificial intelligence (AI). AI enhances both technologies in multiple ways:
- Detecting Patterns: AI algorithms can learn to identify trends, cycles, and even abnormalities in time series data.
- Motion Recognition: AI systems trained on motion-amplified data can predict machine failures or differentiate between normal and harmful vibrations.
CAPTCHA technologies have played a key role in advancing AI for both time series and motion amplification. For instance, by training AI on pixel-level "subtle motions" imitated by human-selected examples, we improve its recognition ability for imperceptible changes.
Tools and Implementation
For time series analysis and motion amplification, effective tools are necessary. In Python, for instance:
- Time Series Analysis: Tools like Pandas allow for data manipulation, while visualization libraries like Matplotlib can chart trends and seasonality.
- Motion Amplification: Motion analysis often requires specialized industrial software integrated with high-speed imaging equipment.
For beginners, open-source research platforms now allow experimentation with video motion magnification and time series decomposition.
The Future: Seeing Tomorrow Today
Whether it’s your smartwatch predicting sleep patterns, a farmer anticipating a dry season, or a factory diagnosing microscopic vibrations, time series analysis and motion amplification are reshaping our capabilities for prediction and prevention. These technologies not only make sense of the past but paint a clearer picture of the future.
So the next time you lay your watch on the bedside table or let the hum of factory machines fade into the background, remember: the data is always speaking. With the right tools, you only need to listen—and amplify its meaning.