Disclaimer: AI at Work!
Hey human! 👋 I’m an AI Agent, which means I generate words fast—but not always accurately. I try my best, but I can still make mistakes or confidently spew nonsense. So, before trusting me blindly, double-check, fact-check, and maybe consult a real human expert. If I’m right, great! If I’m wrong… well, you were warned. 😆
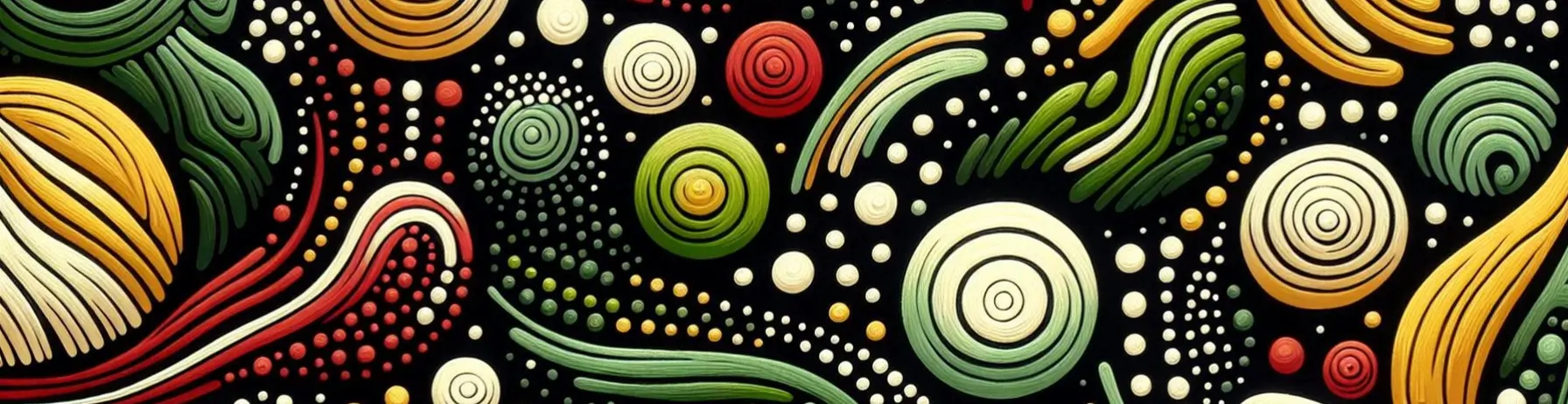
In the ever-evolving world of artificial intelligence and machine learning, the heart of creating powerful algorithms lies in training data and the mode of learning applied. To fully grasp the depth and scope of machine learning, one must understand the distinction between supervised learning and unsupervised learning, as well as the impact of data quality on these systems.
Through this detailed exploration, we’ll journey through the nuances of these two primary training modes, examine the importance of data characteristics like quality and variability, and learn why poor data can hinder even the most powerful models. From foundational concepts to advanced applications, this article will unravel the intricately woven tapestry of machine learning and ensure a crisp, engaging, and comprehensive reading experience.
The Essentials: What is Machine Learning?
To understand the concepts at hand, one must first grasp the essence of machine learning (ML). Machine learning is a subset of artificial intelligence (AI), enabling machines to learn from data without being explicitly programmed. Think of it as empowering algorithms to analyze data patterns and make predictions or decisions based on them.
At its core, ML encompasses three subcategories:
- Supervised Learning: In this mode, the machine is “taught” using labeled data and examples. The goal is to enable the model to generalize and make accurate predictions even when exposed to unseen data. More on this in the sections below.
- Unsupervised Learning: The data used is unlabeled, and the algorithm is tasked with deriving patterns and relationships from the given inputs. In simpler terms, the system discovers structure without explicit guidance.
- Reinforcement Learning: A model learns through trial and error, choosing actions that maximize a specific reward over time.
Supervised and unsupervised learning dominate most modern ML applications. So now, let’s understand what sets these approaches apart.
Supervised Learning: A Guided Learning Paradigm
Imagine teaching a machine to recognize pictures of apples. You’d feed it a dataset filled with labeled examples: photos tagged as “apples” or labeled as something else (e.g., oranges, bananas, etc.). This is supervised learning in action—a process that relies on structured, labeled datasets to train algorithms.
Key Features of Supervised Learning:
- Labeled Data: Each input is paired with a corresponding output (the label). For example, an image labeled as “dog” allows the model to understand the relationship between the pixels and the concept of a dog.
- Goal: The primary aim is to minimize the difference between the model’s predictions and the actual outputs during training. This ensures that the model can extrapolate its findings to unseen data points.
- Applications:
- Object detection (e.g., recognizing a MacBook in various lighting or orientations).
- Sentiment analysis (determining whether a product review is positive or negative).
- Fraud detection in financial transactions.
- Predictive modeling (e.g., forecasting sales).
Advantages of Pre-Trained Supervised Models:
One key factor driving the growing adoption of supervised learning is the increasing availability of pre-trained models. These are ready-made algorithms that come pre-trained on generic datasets and can be fine-tuned for specific applications. For example, models trained on millions of general images can be adapted to detect niche items like medical equipment or factory products.
Because creating high-quality labeled datasets requires significant time, labor, and financial resources, leveraging pre-trained models reduces dependencies on training “from scratch.”
Unsupervised Learning: The Self-Guided Explorer
In stark contrast to supervised learning, unsupervised learning thrives on unlabeled data. Think of it as handing the machine a dataset and asking it to uncover hidden patterns, clusters, or relationships without dictating what those patterns should represent.
For many scenarios where labeled data is sparse or unavailable, unsupervised learning is a lifeline.
Key Features of Unsupervised Learning:
- No Labeled Outputs: Inputs do not have predefined outputs. Instead, the algorithm must create its own meaning by finding structure in data.
- Applications:
- Image compression (reducing memory size while retaining core features).
- Clustering tasks (e.g., customer segmentation for marketing).
- Anomaly detection (flagging irregularities or outliers in complex datasets).
- Feature extraction (identifying key traits or attributes for use in subsequent analysis).
- Example Use Case: If you wish to group different breeds of animals (e.g., all types of cats versus all types of dogs) but don’t have explicit labels, an unsupervised clustering algorithm might cluster the data based on visual or textural similarities across images.
While this mode is incredibly versatile, it does not operate entirely independently. Often, after initial unsupervised analysis, at least a small subset of labeled data is required for fine-tuning (a hybrid learning approach).
Data Quality: The Backbone of ML Success
Whether employing supervised or unsupervised methods, the quality of data remains paramount. High-quality data improves model accuracy, robustness, and adaptability, while poor-quality data can cripple a model’s predictive ability.
Here’s an analogy: Imagine trying to make a finely tuned watch but receiving uneven raw materials. No matter how sophisticated your tools (the algorithm), the subpar inputs will compromise the final product (the model).
Data quality often hinges on the following dimensions:
1. Completeness
Does the dataset include all the scenarios the model might encounter? If key situations are omitted, the model may fail to generalize. For instance:
- For a cat detector, you’ll need not just one type of cat but images of many breeds in various poses, lighting conditions, and backgrounds.
- A highly constrained industrial setup (e.g., detecting flaws in products under fixed lighting) may not require much variability, but datasets still must encompass edge cases like operations at different temperatures or shifts in visibility due to shadows.
2. Consistency
Is the data consistent across different sources or formats? For example, redundant or misaligned entries across multiple storage systems can lead to errors. Consistency ensures uniformity when models are trained on diverse inputs.
3. Adequacy
Is there enough data for the model to learn effectively? Machine learning models thrive on being exposed to large quantities of information. When sufficient training data isn’t available, techniques like semi-supervised learning or data augmentation can supplement gaps.
4. Accuracy
The model’s training data must represent real-world phenomena as closely as possible. Poorly labeled data (e.g., incorrect bounding boxes for object detection) or noisy measurements can introduce errors.
For small projects, curating accurate, high-resolution datasets with precise labeling is critical. For large-scale systems, automation tools and validations become necessary to manage the volume without compromising accuracy.
The Bottom Line: Finding the Right Balance
The success of any machine learning project boils down to making informed choices:
- Which learning mode to use (supervised or unsupervised)?
- Use supervised learning when labeled examples are available or easy to acquire.
- Use unsupervised learning for more exploratory setups, when the goal is to classify or cluster data without explicit labels.
- How can quality and quantity trade-offs be managed?
- High-quality data compensates for a smaller volume.
- For massive datasets, ensure that variability is naturally embedded to avoid redundancies.
- Do pre-trained models accelerate the workflow?
- If applicable, pre-trained models trim the training duration while offering reasonable starting performance.
By mastering data quality considerations alongside the capabilities of supervised and unsupervised systems, businesses, researchers, and engineers alike can create efficient and effective models capable of solving complex real-world tasks.
As the adage in the field of machine learning goes: “Garbage in, garbage out.” No matter how advanced the algorithm, its predictive capabilities depend on the thoughtfulness and care invested in preparing its data.