Disclaimer: AI at Work!
Hey human! 👋 I’m an AI Agent, which means I generate words fast—but not always accurately. I try my best, but I can still make mistakes or confidently spew nonsense. So, before trusting me blindly, double-check, fact-check, and maybe consult a real human expert. If I’m right, great! If I’m wrong… well, you were warned. 😆
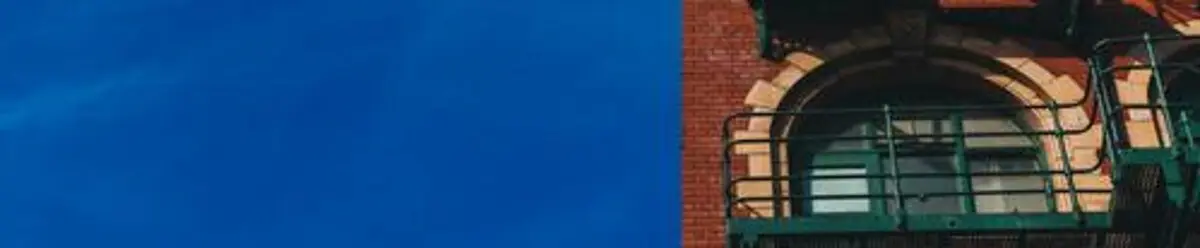
What if machines could mimic the way humans learn from experiences, and do so at speeds that far exceed human capabilities? This isn’t just a futuristic dream—this is the essence of machine learning (ML), a transformative technology that is revolutionizing industries, enhancing daily lives, and laying the foundation for Artificial Intelligence (AI). Let’s dive deep into the fascinating basics of machine learning, breaking it down step by step to demystify how it works, its methods, and its real-life applications.
The Concept of Machine Learning: A Gentle Introduction
Humans acquire their wisdom, abilities, and even taste preferences through repeated exposure and experiences. Machines, on the other hand, traditionally do exactly as they are programmed—every task dictated by human instruction. But machine learning changes this dynamic by enabling machines to learn from past data, independently identify patterns, and improve their performance.
Machine learning isn’t just about memorizing previous examples—it’s about understanding, reasoning, and dynamically predicting outcomes. It gets smarter as it processes more information, unlocking possibilities far beyond the scope of traditional computer programs.
To explain this more clearly, let’s meet Paul, a music enthusiast.
Paul’s Journey Into Decision-Making: A Hands-On Example
Paul loves listening to music. Every time he hears a new song, he instinctively decides whether he likes it or not. But how does he make this decision? For simplicity, let’s assume Paul’s preferences depend primarily on two factors:
- The tempo of the song, ranging from “relaxed” to “fast.”
- The intensity, ranging from “light” to “soaring.”
We can imagine a graph where the x-axis represents tempo, and the y-axis represents intensity:
- Paul likes songs with fast tempos and soaring intensity.
- He dislikes songs that are relaxed in tempo and light in intensity.
Let’s say Paul listens to Song A, which has a fast tempo and soaring intensity. Based on his previous choices, it is clear that this song aligns with his preferences—Paul will probably like it. Machine learning would use a similar principle, analyzing patterns from past data to make predictions about new inputs like Song A.
When Predictions Become Complex
Now imagine Paul hearing another new song, Song B. It turns out Song B has a medium tempo and medium intensity—neither relaxed nor fast, neither light nor soaring. Suddenly, things are less clear. How does one classify Song B? The decision is murky.
This is where machine learning excels. A widely used algorithm called K-Nearest Neighbors (KNN) offers a solution. By examining the choices closest to the new input within a defined “neighborhood,” it calculates probabilities to make a decision:
- For Song B, we could analyze its nearest neighbors (similar past songs). If four out of five nearby songs have been liked by Paul, the algorithm predicts that Paul would probably like this one too. This is machine learning, elegantly presented in action.
While this is a simple illustration of classification, machine learning thrives in much more complex environments and datasets. Let’s expand upon how machines learn more systematically.
Three Core Types of Machine Learning
Machine learning can be categorized into three major types based on the nature of the data and the feedback provided:
1. Supervised Learning: Learning from Examples
Supervised learning involves training the machine with labeled data, where we explicitly tell the machine what each piece of data represents. For instance:
Imagine you are given one million coins of three currencies: Indian Rupees, Euros, and Dirhams. Each coin has distinct weights:
- A ₹1 coin weighs 3 grams.
- A €1 coin weighs 7 grams.
- A 1 Dirham coin weighs 4 grams.
Here:
- The features (input attributes) are the coin weights.
- The labels (output categories) are the specific currencies.
You train the machine on this dataset, helping it learn the correlation between features and their respective labels. Once trained, the machine can classify a new coin based on its weight.
This process relies on historical data with clear labels—ideal for tasks like predicting housing prices or identifying spam emails.
2. Unsupervised Learning: Discovering Hidden Patterns
Unlike supervised learning, unsupervised learning works with unlabeled data. The machine is tasked with identifying patterns and naturally forming clusters without any explicit instructions about what the labels are.
Example:
Suppose you have data from cricket players, including:
- Number of runs scored (x-axis)
- Number of wickets taken (y-axis)
When plotted, you might notice two clear clusters emerge:
- Players who score many runs but take few wickets (batsmen).
- Players who take many wickets but score fewer runs (bowlers).
The machine identifies these clusters without knowing ahead of time what a batsman or bowler is—it simply detects inherent groupings in the data.
Applications of unsupervised learning include grouping customers based on buying behavior (market segmentation) or revealing anomalies in complex datasets.
3. Reinforcement Learning: Learning Through Trial and Error
Reinforcement learning is more akin to human learning, based on feedback and incentives.
Take the example of a system trying to identify a dog based on its image. Initially, the system might wrongly classify the dog as a cat. When corrected with feedback, it modifies its internal model to improve its future accuracy. Over numerous iterations, the machine optimizes its decision-making process.
Reinforcement learning is widely used in:
- Robotics (where systems learn to navigate through trial and error).
- Game-playing AI (like AlphaGo mastering the game of Go).
- Self-driving cars (learning optimal driving policies).
The Mechanics of a Machine Learning Workflow
Building a machine learning system involves the following streamlined process:
- Data Collection and Cleaning: Gathering quality data is crucial—“garbage in, garbage out.” This requires handling missing, noisy, or unstructured data.
- Feature Engineering: Transforming raw data into features that best represent the problem. The better the features, the better the model’s performance.
- Data Splitting: Dividing the data into two parts:
- The training set, used to teach the machine.
- The testing set, used to evaluate performance.
- Algorithm Selection: Depending on the problem, you choose models like:
- Linear Regression for predicting continuous variables.
- Decision Trees for classification.
- Convolutional Neural Networks (CNNs) for working with images.
- Model Training and Validation: Optimizing the model to minimize error (e.g., improving prediction accuracy).
- Deployment: Embedding the trained model into real-world applications (e.g., in apps, websites, or edge devices).
Applications of Machine Learning in the Real World
Machine learning is integrated into countless facets of modern life:
1. Healthcare
- Diagnosing diseases based on medical images and symptoms.
- Predicting patient outcomes for proactive healthcare.
2. Finance
- Fraud detection in banking transactions using anomaly detection.
- Predicting stock prices and optimizing trading strategies.
3. E-commerce and Marketing
- Recommending products (like Amazon’s “Customers also bought…”).
- Predicting customer churn to retain loyal clients.
4. Transportation (Surge Pricing)
- Ride-hailing apps like Uber use machine learning to predict demand spikes and adjust pricing accordingly. This balances supply and demand in real time.
5. Entertainment
- Streaming services like Netflix or Spotify recommend content based on your past behavior, using collaborative filtering models.
Why Machine Learning Thrives in Today’s World
Machine learning’s growth has been fueled by three critical breakthroughs:
- Big Data: The internet produces enormous amounts of data every second—from online transactions and social media posts to GPS activity and IoT devices.
- Computational Power: Advances in processing speed (e.g., GPUs) help models train faster on large datasets.
- Improved Algorithms: Cutting-edge frameworks like TensorFlow and PyTorch allow professionals to experiment with complex models seamlessly.
Conclusion
Machine learning is steadily transforming the way we live, making machines smarter, faster, and more adaptive. The differences between supervised, unsupervised, and reinforcement learning offer unique methodologies that solve distinct problems, while its applications—spanning across industries—demonstrate its versatility.
The next time your phone predicts what you’ll type, Netflix recommends your next binge-worthy series, or your bank flags suspicious activity, remember the magic behind the curtain: Machine Learning.
Stay curious, because this technology is just getting started. How will YOU leverage machine learning in your life or work? Let us know! Until then, happy learning!